Healthcare Analytics
By Govind Kumar
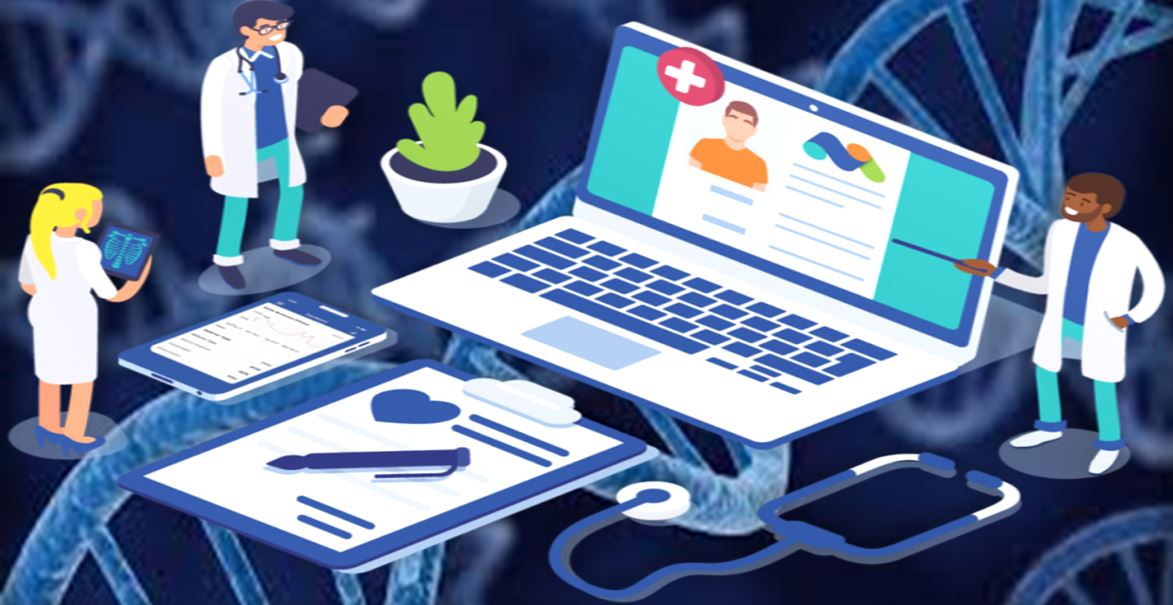
The 21st century has been an era of data-driven decisions and nowhere is this more useful than in healthcare. Healthcare industry generates huge amount of data and this offers a great opportunity to intelligently use the data to make a critical difference to life expectancy.
Machine learning algorithms enables healthcare professionals in better decision making, identifying trends and most importantly in improving the efficiency of research and clinical trials. There are many areas in healthcare where machine learning plays a role already and the importance of AI in healthcare will significantly increase in the future. The technology will only mature over time.
Broadly speaking, we can identify three areas where ML is being used in healthcare right now:
- Perception tasks – tasks requiring skills of perception such as vision or hearing etc.
- Diagnostic assistance
- Treatment procedures
Perception Tasks: Deep neural networks have boosted the performance of computers at perception tasks to previously unimaginable levels. It is very likely that the performance of machine learning algorithmsin areas where perception tasks are central, will improve further,thus enhancing the productivity of physicians and improving the level of healthcare. For example, in radiology, where the task of the physician is to diagnose a patient using medical imaging, computers have been taught to identify pathologies from such images at either a comparable or even higher level than human doctors.
Diagnostic Assistance: Machine learning is being used to augment the physician’s capacity by looking at the total available data on the patient and making recommendations based on this information. Diagnostic assistance is used for assisting the physicians in real time by either data retrieval or diagnosis recommendations. A prediction service for identifying at-risk patients is also being offered.
Treatment process: Being an important part of healthcare, improvements in this process produces gains in both quality and cost of care. For this end, a branch of data science called process mining has been utilized with quite a bit of success.
Machine Learning is changing the current healthcare in many ways, ranging from early and adaptive diagnosis to high volume processing of data, collaborative analysis of dataand highly personalized medicine. In the past, predictions were made with equation-based mathematical methods and tools which gave very low accuracy rate. But with the recent development in technologies such as big data and machine learning, it is possible to get more accurate results for disease prediction.
Machine Learning and Cancer detection
In the last two decades, a variety of different ML techniques and feature selection algorithms have been widely applied to disease prognosis and prediction.When dealing with cancer prognosis/prediction, one is concerned with three predictive tasks:
- the prediction of cancer susceptibility (risk assessment)
- the prediction of cancer recurrence and
- the prediction of cancer survival.
In the first two cases one is trying to find:
- the likelihood of developing a type of cancer and
- the likelihood of re-developing a type of cancer after complete or partial remission.
In the last case, the prediction of a survival outcome such as disease-specific survival or overall survival, after cancer diagnosis or treatment, is the main objective.
The prediction of cancer outcome usually refers to the cases of
- life expectancy
- survivability
- progression and
- treatment sensitivity
Machine learning techniques like ANNs (Artificial Neural network) and DTs (Decision Tree) have been used in the past for cancer detection. In recent times, other supervised learning techniques, namely SVMs (Support Vector Machine) and BNs (Bayesian Network) are being used in cancer prediction and prognosis. All of these classification algorithms are being used to address a wide range of problems in cancer.The analysis of big data can be particularly useful for improving cancer patient’s care and research in oncology. In radiology there are many systems that allow recognizing the pathology in the picture, reconstructing 3D image from 2D images. There are also expert systems that allowmaking clinical decisions in radiology.
In future, machine learning will not only help to forecast potential issues and diseases, but also suggest what steps a patient should take and how medical staff should react.
Do you want to try SeaportAI’sMachine Learning based disease prediction tool ? Click here